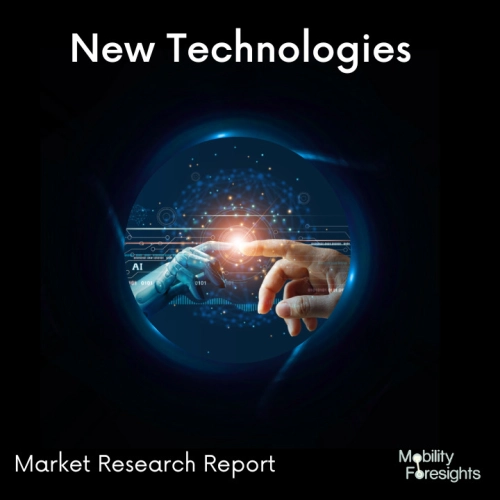
- Get in Touch with Us
Last Updated: Apr 27, 2025 | Study Period: 2024-2030
Artificial Intelligence (AI) hardware has emerged as a transformative force, revolutionizing the landscape of computing and enabling remarkable advancements in various industries. AI hardware refers to specialized hardware components and systems designed to accelerate AI workloads, such as machine learning and deep learning tasks.
These components are tailored to address the unique computational requirements of AI algorithms, offering substantial improvements in speed, efficiency, and performance compared to traditional computing architectures.
AI hardware encompasses a diverse range of technologies, each optimized for specific AI tasks. One of the cornerstones of AI hardware is the Graphics Processing Unit (GPU). Originally designed for rendering graphics, GPUs have been repurposed for AI due to their parallel processing capabilities.
GPUs excel in handling the matrix calculations fundamental to many machine learning algorithms, making them a powerful choice for training complex models. Another key player in AI hardware is the Field-Programmable Gate Array (FPGA). FPGAs offer reconfigurability, allowing developers to tailor hardware circuits to match the specific requirements of an AI application. This adaptability makes FPGAs well-suited for applications that demand high performance with low latency, like real-time analytics and inference tasks.
Application-Specific Integrated Circuits (ASICs) represent a more specialized category of AI hardware.These chips are designed from the ground up for AI workloads, resulting in unmatched efficiency and speed. ASICs can be further divided into two categories: Training ASICs and Inference ASICs.
Training ASICs are optimized for training large AI models, while Inference ASICs focus on rapidly executing pre-trained models, making them suitable for applications like autonomous vehicles and voice assistants. Neuromorphic hardware is an emerging frontier in AI technology inspired by the human brain's neural architecture. These chips, often referred to as neuromorphic chips, aim to mimic the brain's parallel and energy-efficient computation. They hold the potential to revolutionize AI by enabling low-power, high-speed, and highly adaptive processing, ideal for edge computing and robotics.
Quantum computing, while still in its experimental stages, has also entered the AI hardware conversation.Quantum computers leverage the principles of quantum mechanics to perform computations at speeds inconceivable by classical computers. They have the potential to revolutionize AI by solving highly complex problems that are currently infeasible to tackle.
AI hardware's significance goes beyond its technological prowess; it drives groundbreaking applications across industries. In healthcare, AI-powered hardware accelerates medical imaging analysis, drug discovery, and personalized treatment plans. Industries like finance benefit from AI's data analysis capabilities for fraud detection, risk assessment, and algorithmic trading.
Autonomous vehicles heavily rely on AI hardware for real-time decision-making, enabling safe navigation.Furthermore, AI hardware plays a pivotal role in optimizing energy consumption in smart grids, reducing waste in manufacturing processes through predictive maintenance, and enhancing customer experiences in e-commerce. The demand for efficient AI hardware has spurred innovation and competition among tech giants and startups alike.Companies are investing heavily in research and development to create more powerful and energy-efficient solutions. This drive has led to remarkable breakthroughs in chip architecture, memory systems, and interconnect technologies.
However, AI hardware is not without its challenges.The rapid evolution of AI algorithms requires hardware that can keep up with the increasing complexity and computational demands.Cooling and power consumption are also critical concerns, particularly in data centers that house massive clusters of AI hardware. Additionally, ensuring compatibility and optimization between software frameworks and hardware is an ongoing effort. In conclusion, AI hardware stands as a testament to the symbiotic relationship between technological advancements and human progress.
Its evolution has enabled AI to transcend theoretical concepts, powering real-world applications with profound implications. From GPUs and FPGAs to neuromorphic chips and quantum computers, AI hardware continues to shape industries, push computational boundaries, and drive innovation at an unprecedented pace. As AI's influence on society expands, so too will the capabilities of AI hardware, forging a future where machines augment human potential across every facet of life.
The Global Ai Hardware Market accounted for $XX Billion in 2023 and is anticipated to reach $XX Billion by 2030, registering a CAGR of XX% from 2024 to 2030.
Google Cloud TPUv4 Pods: Google Cloud TPUv4 Pods are a new generation of custom-designed machine learning accelerators that are up to 18 times faster than the previous generation. They are based on the 7nm process and can deliver up to 480 petaflops of performance. TPUv4 Pods are designed for large-scale deep-learning training and inference workloads.NVIDIA Grace Hopper Superchip: NVIDIA Grace Hopper Superchip is a new AI accelerator that is designed to power the next generation of supercomputers.
It is based on the Hopper GPU architecture and can deliver up to 1.8 exaflops of performance. Grace Hopper Superchip is designed for training and deploying large language models, natural language processing, and other AI workloads.
Graphcore Bow Arrow: Graphcore Bow Arrow is a new AI accelerator that is designed to accelerate graph neural network (GNN) workloads. It is based on the Colossus AI chip architecture and can deliver up to 100 teraflops of performance per chip.Bow Arrow is designed for training and deploying GNNs for applications such as natural language processing, computer vision, and drug discovery.
Habana Gaudi: Habana Gaudi is a new AI accelerator that is designed to accelerate the training and inference of large language models.It is based on the Gaudi chip architecture and can deliver up to 40 teraflops of performance per chip.Gaudi is designed for applications such as natural language processing, machine translation, and text summarization.
North America
The North American market, particularly the USA, will be one of the prime markets for (AI Hardware Market) due to the nature of industrial automation in the region, high consumer spending compared to other regions, and the growth of various industries, mainly AI, along with constant technological advancements. The GDP of the USA is one of the largest in the world, and it is home to various industries such as Pharmaceuticals, Aerospace, and Technology. The average consumer spending in the region was $72K in 2023, and this is set to increase over the forecast period. Industries are focused on industrial automation and increasing efficiency in the region. This will be facilitated by the growth in IoT and AI across the board. Due to tensions in geopolitics, much manufacturing is set to shift towards the USA and Mexico, away from China. This shift will include industries such as semiconductors and automotive.
Europe
The European market, particularly Western Europe, is another prime market for (AI Hardware Market) due to the strong economic conditions in the region, bolstered by robust systems that support sustained growth. This includes research and development of new technologies, constant innovation, and developments across various industries that promote regional growth. Investments are being made to develop and improve existing infrastructure, enabling various industries to thrive. In Western Europe, the margins for (AI Hardware Market) are higher than in other parts of the world due to regional supply and demand dynamics. Average consumer spending in the region was lower than in the USA in 2023, but it is expected to increase over the forecast period.
Eastern Europe is anticipated to experience a higher growth rate compared to Western Europe, as significant shifts in manufacturing and development are taking place in countries like Poland and Hungary. However, the Russia-Ukraine war is currently disrupting growth in this region, with the lack of an immediate resolution negatively impacting growth and creating instability in neighboring areas. Despite these challenges, technological hubs are emerging in Eastern Europe, driven by lower labor costs and a strong supply of technological capabilities compared to Western Europe.
There is a significant boom in manufacturing within Europe, especially in the semiconductor industry, which is expected to influence other industries. Major improvements in the development of sectors such as renewable energy, industrial automation, automotive manufacturing, battery manufacturing and recycling, and AI are poised to promote the growth of (AI Hardware Market) in the region.
Asia
Asia will continue to be the global manufacturing hub for (AI Hardware Market) over the forecast period with China dominating the manufacturing. However, there will be a shift in manufacturing towards other Asian countries such as India and Vietnam. The technological developments will come from China, Japan, South Korea, and India for the region. There is a trend to improve the efficiency as well as the quality of goods and services to keep up with the standards that are present internationally as well as win the fight in terms of pricing in this region. The demand in this region will also be driven by infrastructural developments that will take place over the forecast period to improve the output for various industries in different countries.
There will be higher growth in the Middle East as investments fall into place to improve their standing in various industries away from petroleum. Plans such as Saudi Arabia Vision 2030, Qatar Vision 2030, and Abu Dhabi 2030 will cause developments across multiple industries in the region. There is a focus on improving the manufacturing sector as well as the knowledge-based services to cater to the needs of the region and the rest of the world. Due to the shifting nature of fossil fuels, the region will be ready with multiple other revenue sources by the time comes, though fossil fuels are not going away any time soon.
Africa
Africa is expected to see the largest growth in (AI Hardware Market) over the forecast period, as the region prepares to advance across multiple fronts. This growth aligns with the surge of investments targeting key sectors such as agriculture, mining, financial services, manufacturing, logistics, automotive, and healthcare. These investments are poised to stimulate overall regional growth, creating ripple effects across other industries as consumer spending increases, access to products improves, and product offerings expand. This development is supported by both established companies and startups in the region, with assistance from various charitable organizations. Additionally, the presence of a young workforce will address various existing regional challenges. There has been an improvement in political stability, which has attracted and will continue to attract more foreign investments. Initiatives like the African Continental Free Trade Area (AfCFTA) are set to facilitate the easier movement of goods and services within the region, further enhancing the economic landscape.
RoW
Latin America and the Oceania region will showcase growth over the forecast period in (AI Hardware Market). In Latin America, the focus in the forecast period will be to improve their manufacturing capabilities which is supported by foreign investments in the region. This will be across industries mainly automotive and medical devices. There will also be an increase in mining activities over the forecast period in this region. The area is ripe for industrial automation to enable improvements in manufacturing across different industries and efficiency improvements. This will lead to growth of other industries in the region.
Margin Comparison (Highest to lowest) | Region | Remarks |
1 | Europe | The supply chain demands and the purchasing power in the region enable suppliers to extradite a larger margin from this region than other regions. This is for both locally manufactured as well as imported goods and services in the region. |
2 | North America | Due to the high spending power in this region, the margins are higher compared to the rest of the world, but they are lower than Europe as there is higher competition in this region. All the suppliers of goods and services target USA as a main market thereby decreasing their margins compared to Europe |
3 | Asia | Lower purchasing power, coupled with higher accessibility of services in this regions doesnât enable suppliers to charge a high margin making it lower than Europe and North America. The quality of goods and services are also affected due to this aspect in the region |
4 | Africa and ROW | The margins are the lowest in this region, except for Australia and New Zealand as the countries in this region donât have much spending power and a large portion of the products and services from this area is exported to other parts of the world |
Sl no | Topic |
1 | Market Segmentation |
2 | Scope of the report |
3 | Abbreviations |
4 | Research Methodology |
5 | Executive Summary |
6 | Introduction |
7 | Insights from Industry stakeholders |
8 | Cost breakdown of Product by sub-components and average profit margin |
9 | Disruptive Innovation in the Industry |
10 | Technology Trends in the Industry |
11 | Consumer trends in the industry |
12 | Recent Production Milestones |
13 | Component Manufacturing in the US, EU and China |
14 | COVID-19 impact on overall market |
15 | COVID-19 impact on Production of components |
16 | COVID-19 impact on the point of sale |
17 | Market Segmentation, Dynamics and Forecast by Geography, 2024-2030 |
18 | Market Segmentation, Dynamics and Forecast by Product Type, 2024-2030 |
19 | Market Segmentation, Dynamics and Forecast by Application, 2024-2030 |
20 | Market Segmentation, Dynamics and Forecast by End Use, 2024-2030 |
21 | Product installation rate by OEM, 2023 |
22 | Incline/Decline in Average B-2-B selling price in the past 5 years |
23 | Competition from substitute products |
24 | Gross margin and average profitability of suppliers |
25 | New product development in the past 12 months |
26 | M&A in the past 12 months |
27 | Growth strategy of leading players |
28 | Market share of vendors, 2023 |
29 | Company Profiles |
30 | Unmet needs and opportunities for new suppliers |
31 | Conclusion |
32 | Appendix |