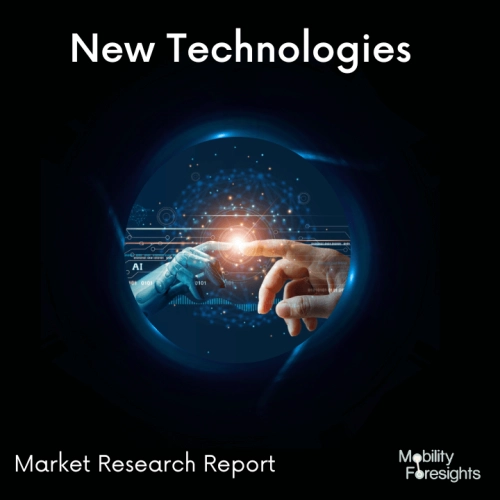
- Get in Touch with Us
Last Updated: Apr 25, 2025 | Study Period: 2024-2030
The basis of most of these technologies is framed by big data and analytics, as enormous volumes of information are gathered by sensor technologies, which have been analysed and also used to change the car industry, promote mechanisation, and enhance mechanization.
Inside the near future, information examination will most likely be the primary driving force behind vehicle technology and progress in this field.
The advancement of data analytics in the car industry is likely to have a significant impact, since vehicles will be able to communicate, collaborate data, and manoeuvre without any need for operator interaction while supplying high levels of information.
Through use of big data is also assisting automakers in significantly lowering costs by assisting them in researching new ways and employing materials that are likely to be obsolete.
Advanced analytics provides manufacturers with a more in-depth understanding of their customers by studying a wide range of data points.
Automobile manufacturers can identify critical experiences for developing targeted advertising initiatives in order to cut costs and provide more notable brand exposure. A linked automobile features bidirectional data exchange with equipment both inside and outside the vehicle.
It is critical for a person inside a car to have access to data, such as news or merely audio on the move, maps, traffic advisories, weather forecasts, and a variety of other reliable details.
Risks can be mitigated, as well as the car's motorist can arrange their journey appropriately. Most importantly, analysing data collected by an automobile boosts dependability and durability while also giving comfort to the driver. Prescriptive modelling is among the most essential types of big data and analytics.
The advanced analytics industry is now in its early stages, with minimal competitiveness. Leading companies use new product launches and product innovation as major tactics to attract more customers as well as diversification.
Big data analytics inside the automotive industry is driven by increased awareness about the benefits of big data and analytics, advancements in big data processing, unrelenting investment by online giants and manufacturing leaders, and an increase in data quantity.
Nevertheless, a scarcity of analytical professionals and ambiguous big data approaches are projected to stifle the expansion of big data analytics in the automotive business.
The advent of cloud computing opens up new avenues for development. Considering system adaptability and administration, as well as data protection, are two of the most important considerations.
Big Data is quickly gaining momentum across a wide range of vertical industries, owing to the growth of real-time and historical data from sources such as linked devices, online, social networking sites, analytics, application logs, and operational applications.
Big Data has already developed a variety of uses in the automobile sector, spanning from concept development and installation to predicting vehicle maintenance and automated driving.
Additionally, big data analytics has assisted automotive manufacturers in increasing their marketing and advertising effectiveness. It has also aided in the adoption of utilities such as predictive maintenance and service schedules, which has enhanced its operations.
It has also benefited automotive vendors in refining the acquisition system and making it much more cost-effective by evaluating data for estimating.
The Global Automotive Big Data Market can be segmented into following categories for further analysis.
As technology solutions, implementations, and systems running on advances with everything from detectors to artificial intelligence to business intelligence tools reshape the vehicle, the environment is witnessing a fairly constant surge of new performers as well as the continued evolution of the roles played by key stakeholders and the balance of power among them.
The increasing partnership between manufacturers and development companies is of special relevance. Analytics enables the merging of data depending on the type, which could include machine-readable databases or unstructured information also including films, recorded conversations, or documents.
When done correctly, the effects are spectacular. This has been accepted from a distance, inconsistently, and sometimes not clearly addressed in the automobile industry.
The sheer volume of data presently available might be overwhelming. Within the automotive industry, the supply chain requirements and quality control requirements form the critical points of adherence to be made for continued services operations.
Integrated supply chain intelligence is gradually allowing the international automotive sector to break away from traditional point-in-time snapshot and toward real-time accessing data, which pushes assessment and transparency to participants inside an institution and beyond the distribution network.
Using cloud infrastructure and Big Data technologies will become much more mainstream. Predictive assurance analytics capabilities currently available enable control procedures to be discovered and corrected very earlier onwards, allowing standard management teams to handle both customer loyalty and budgetary control considerations more effectively.
Large amounts of data can now be processed by sophisticated computing systems. It gathers information and provides numerous analytical tools, allowing me to spot possible flaws ahead of time and provide possibilities to design appropriate counterproductive fixes. The use of such predictive quality analytics systems will have a positive impact.
Big data enables the automobile manufacturing sector to acquire data through Enterprise systems in order to integrate information from the various corporate functional departments and related stakeholders.
The automobile sector is preparing for Industry 4.0 also with the introduction of industrial IoT, a networked system, and M2M communications. Sensors, RFIDs, barcode scanners, and robotics are already commonplace on the production floor.
For many industrialised nations, such as the United Kingdom, the consequences of its automotive manufacturing base and importance as a significant revenue territory for automakers are evident; connection to customers and their vehicles may have a powerful effect on the distribution network.
Red hat is a large-scale deployer of the automated big data analytics requirements of the automotive sectors of the global market. Red Hat OpenShift is indeed a market-leading enterprise Kubernetes system that enables a cloud-like environment wherever it may be installed.
Red Hat OpenShift allows users to find wherever businesses create, distribute, and execute services, whether it's in the cloud, on-premises, or at the edges, while providing a consistent experience.
Red Hat OpenShift's completely automated management and self-service deployment for engineers enable organizations to collaborate more effectively as innovations migrate from research to production.
This is a Kubernetes container platform designed for an accessible hybrid cloud architecture. It offers a standardised application framework for managing hybrid cloud, multi cloud, or edge deployments.
AI/ML, Java, data analytics, databases, and other challenging applications are all supported. With the extensive ecosystem of technology companies, designers can automate deployment and life-cycle administration.
Q Burst is making its strides towards better and optimised big data analytics and operability of data for visual requirements on the global level of market. Hadoop, with its distributed file system and MapReduce parallel computing engine, provides a robust big data platform for enormous data processing.
Hadoop, which began as a batch processing system, has developed to allow real-time computing through the use of tools such as Storm and Spark. Along with this, Spark is mostly used for parallel programming.
Featuring 100x in-memory and 10x disc speed, Spark's in-memory stream information processing outperforms Hadoop's MapReduce paradigm. The processing approach of Spark is suited for real-time interactive searching, graphing computational evaluation, and machine learning.
NiFi, which is centered on flow-based computing ideas, automates data flow management, and assists in addressing difficulties that frequently develop while processing data from numerous business systems. Its simple graphical interface makes it simple to use.
Sl no | Topic |
1 | Market Segmentation |
2 | Scope of the report |
3 | Abbreviations |
4 | Research Methodology |
5 | Executive Summary |
6 | Introduction |
7 | Insights from Industry stakeholders |
8 | Cost breakdown of Product by sub-components and average profit margin |
9 | Disruptive innovation in theIndustry |
10 | Technology trends in the Industry |
11 | Consumer trends in the industry |
12 | Recent Production Milestones |
13 | Component Manufacturing in US, EU and China |
14 | COVID-19 impact on overall market |
15 | COVID-19 impact on Production of components |
16 | COVID-19 impact on Point of sale |
17 | Market Segmentation, Dynamics and Forecast by Geography, 2024-2030 |
18 | Market Segmentation, Dynamics and Forecast by Product Type, 2024-2030 |
19 | Market Segmentation, Dynamics and Forecast by Application, 2024-2030 |
20 | Market Segmentation, Dynamics and Forecast by End use, 2024-2030 |
21 | Product installation rate by OEM, 2023 |
22 | Incline/Decline in Average B-2-B selling price in past 5 years |
23 | Competition from substitute products |
24 | Gross margin and average profitability of suppliers |
25 | New product development in past 12 months |
26 | M&A in past 12 months |
27 | Growth strategy of leading players |
28 | Market share of vendors, 2023 |
29 | Company Profiles |
30 | Unmet needs and opportunity for new suppliers |
31 | Conclusion |
32 | Appendix |