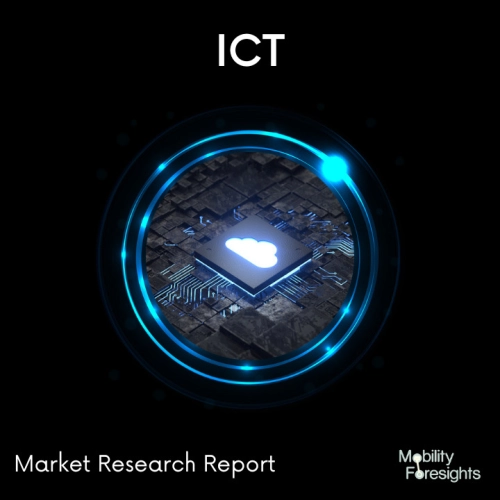
- Get in Touch with Us
Last Updated: Apr 25, 2025 | Study Period: 2024-2030
The method known as "Human Pose Estimation" uses computer vision to recognize and categories particular places on the human body.
These points serve as a representation of a person's limbs and joints for the purpose of determining the angle of flexion and, er, estimating human position.
The portion of the process where the camera cannot see the actual area of contact with the product is handled by human pose estimation.
Therefore, in this instance, the HPE model examines the posture of customers' hands and heads to determine whether they pulled the goods from the shelf or left it there.
The Global Human Pose Estimation Camera market accounted for $XX Billion in 2023 and is anticipated to reach $XX Billion by 2030, registering a CAGR of XX% from 2024 to 2030.
Although it is a difficult task, multi-person 3D posture estimation for a fisheye camera with absolute depths has practical uses, particularly for video surveillance.
To the best of their knowledge, no one has yet looked at this issue, hence there aren't any current applications.
To estimate absolute 3D human poses, this method uses two branches: (1) a 2D to 3D lifting module to forecast root-relative 3D human poses; and (2) A root regression module (Hoot Net) to calculate the positions of the absolute roots in the camera coordinate.
In order to reduce the negative effects of image distortions on 3D pose estimate and further regularize prediction absolute 3D poses, we present a fisheye re-projection module without employing ground-truth camera parameters to connect two branches.
Particularly, fisheye cameras will have a bigger field of vision and more significant distortion factors. The inference of several 3D poses from fisheye photos is necessary for many of these applications.
The majority of existing techniques, however, concentrate on 3D posture estimation from images taken by a perspective camera, and this problem has not been researched.
In this way, their method accounts for visual distortions while estimating multi-person 3D postures, and anticipated absolute depths are further regularized.
In particular, they use a learning-based approach to estimate camera parameters, avoiding the need for ground-truth camera parameters.
Sl no | Topic |
1 | Market Segmentation |
2 | Scope of the report |
3 | Abbreviations |
4 | Research Methodology |
5 | Executive Summary |
6 | Introduction |
7 | Insights from Industry stakeholders |
8 | Cost breakdown of Product by sub-components and average profit margin |
9 | Disruptive innovation in the Industry |
10 | Technology trends in the Industry |
11 | Consumer trends in the industry |
12 | Recent Production Milestones |
13 | Component Manufacturing in US, EU and China |
14 | COVID-19 impact on overall market |
15 | COVID-19 impact on Production of components |
16 | COVID-19 impact on Point of sale |
17 | Market Segmentation, Dynamics and Forecast by Geography, 2024-2030 |
18 | Market Segmentation, Dynamics and Forecast by Product Type, 2024-2030 |
19 | Market Segmentation, Dynamics and Forecast by Application, 2024-2030 |
20 | Market Segmentation, Dynamics and Forecast by End use, 2024-2030 |
21 | Product installation rate by OEM, 2023 |
22 | Incline/Decline in Average B-2-B selling price in past 5 years |
23 | Competition from substitute products |
24 | Gross margin and average profitability of suppliers |
25 | New product development in past 12 months |
26 | M&A in past 12 months |
27 | Growth strategy of leading players |
28 | Market share of vendors, 2023 |
29 | Company Profiles |
30 | Unmet needs and opportunity for new suppliers |
31 | Conclusion |
32 | Appendix |