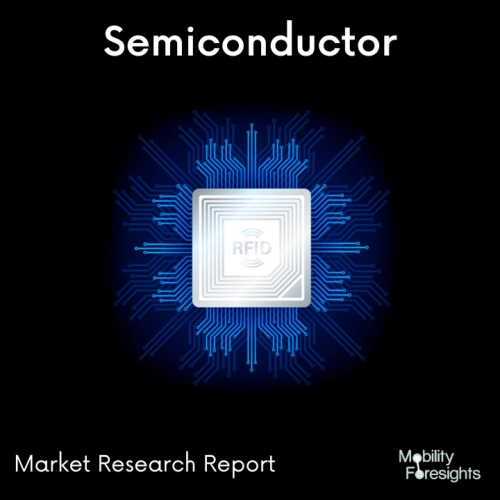
- Get in Touch with Us
Last Updated: Apr 25, 2025 | Study Period: 2024-2030
Field Programmable Gate Arrays (FPGAs) are semiconductor devices that are rapidly becoming integral to the development of data-driven applications.
They offer the potential for greater processing performance than traditional processors, while also providing flexibility in the design of custom circuits.
FPGAs offer many advantages over traditional processors, such as low power consumption, high clock speed, and programmable logic cells.
Recent advances in machine learning have made it possible to effectively use FPGAs to develop data-driven applications.
By leveraging the power of machine learning algorithms, FPGAs can be used to identify patterns in data and make decisions based on those patterns.
This makes FPGAs an ideal platform for applications such as computer vision, natural language processing, and speech recognition.
The use of machine learning algorithms on FPGAs has several advantages. First, FPGAs are highly scalable, allowing for the development of large and complex data-driven applications.
Second, FPGAs are highly energy-efficient compared to traditional processors, which reduces the cost of running data-driven applications. Lastly, machine learning algorithms can be easily adapted to FPGAs since they are reprogrammable.
In summary, the combination of machine learning algorithms and FPGAs is an effective way to develop data-driven applications.
FPGAs offer the potential for high performance, low power consumption, and scalability, while machine learning algorithms provide the ability to identify patterns in data and make decisions based on those patterns.
As a result, FPGA-based machine learning is becoming increasingly popular in the development of data-driven applications.
The Global Machine Learning-Based FPGA market accounted for $XX Billion in 2023 and is anticipated to reach $XX Billion by 2030, registering a CAGR of XX% from 2024 to 2030.
Xilinx is one of the leading companies in this space, offering its Versal AI Core series. This product uses a combination of a neural network, convolutional neural network, and recurrent neural network to optimize FPGA designs.
It also supports a wide range of AI-based applications, such as natural language processing and computer vision. The Versal AI Core series is designed to take advantage of Xilinx's high-performance FPGA and embedded processing platforms, providing a powerful, low-power platform that can handle a range of AI workloads.
Intel has also recently launched its FPGA-based product series, the Intel Programmable Acceleration Card (PAC).
This product combines a range of Intel's technologies, including an FPGA and a range of accelerators, such as the Intel Movidius Neural Compute Stick (NSC).
The Intel PAC is designed to accelerate AI workloads, and is ideal for applications such as deep learning, computer vision, and natural language processing.
Altera, a subsidiary of Intel, has also recently released its own FPGA-based product, the Stratix 10 FPGA. This product is designed to provide high-performance, low-power FPGA for applications such as deep learning, computer vision, and natural language processing. It also supports a range of Altera technologies, such as the OpenCL and OpenVX frameworks.
Sl no | Topic |
1 | Market Segmentation |
2 | Scope of the report |
3 | Abbreviations |
4 | Research Methodology |
5 | Executive Summary |
6 | Introduction |
7 | Insights from Industry stakeholders |
8 | Cost breakdown of Product by sub-components and average profit margin |
9 | Disruptive innovation in the Industry |
10 | Technology trends in the Industry |
11 | Consumer trends in the industry |
12 | Recent Production Milestones |
13 | Component Manufacturing in US, EU and China |
14 | COVID-19 impact on overall market |
15 | COVID-19 impact on Production of components |
16 | COVID-19 impact on Point of sale |
17 | Market Segmentation, Dynamics and Forecast by Geography, 2024-2030 |
18 | Market Segmentation, Dynamics and Forecast by Product Type, 2024-2030 |
19 | Market Segmentation, Dynamics and Forecast by Application, 2024-2030 |
20 | Market Segmentation, Dynamics and Forecast by End use, 2024-2030 |
21 | Product installation rate by OEM, 2023 |
22 | Incline/Decline in Average B-2-B selling price in past 5 years |
23 | Competition from substitute products |
24 | Gross margin and average profitability of suppliers |
25 | New product development in past 12 months |
26 | M&A in past 12 months |
27 | Growth strategy of leading players |
28 | Market share of vendors, 2023 |
29 | Company Profiles |
30 | Unmet needs and opportunity for new suppliers |
31 | Conclusion |
32 | Appendix |