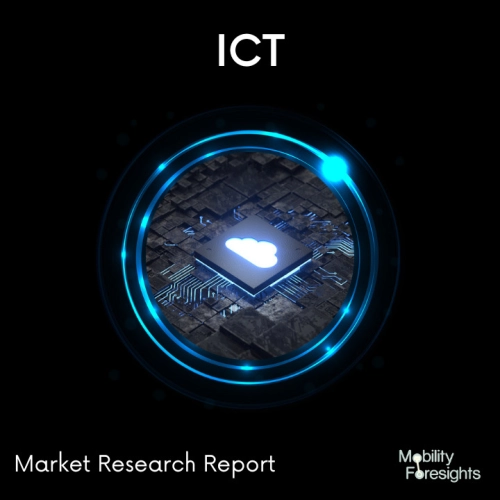
- Get in Touch with Us
Last Updated: Apr 25, 2025 | Study Period: 2023-2030
A new class of microprocessor called a vision processing unit is emerging. A VPU is a particular kind of AI accelerator that is made to speed up tasks involving machine vision. The ability of vision processing units to execute machine vision algorithms like CNN, SIFT, and comparable ones sets them apart from video processing units (which are designed specifically for video encoding and decoding).
The emphasis is more on on-chip dataflow between numerous parallel execution units with scratchpad memory, similar to a manycore DSP, and they may incorporate direct interfaces to take data from cameras. Nonetheless, similar to video processing units, they might prioritise low precision fixed point arithmetic for image processing.
They differ from GPUs in that VPUs are optimised for performance per watt, but GPUs primarily concentrate on absolute performance. GPUs have specialised hardware for rasterization and texture mapping, and its memory architecture is tuned for manipulating bitmap images in off-chip memory.
Robotics, the internet of things, smart cameras, new digital camera classes for virtual reality and augmented reality, and incorporating machine vision acceleration into smartphones and other mobile devices are the target markets. Programmable vision computer enables easy access to computing hardware that was previously only accessible through a variety of occasionally conflicting API.
The Global Programmable vision computer market accounted for $XX Billion in 2022 and is anticipated to reach $XX Billion by 2030, registering a CAGR of XX% from 2023 to 2030.
The NVIDIA Programmable vision computer software library for computer vision and image processing supports algorithms on CPUs, GPUs, programmable vision accelerators, Video and Image Compositors, and Optical Flow Accelerators, among other computing engines.
In order to achieve high picture throughput and simple OpenCV interoperability, Programmable vision computer optimises the algorithms on both NVIDIA Jetson modules and x86 devices with discrete GPUs. It also has an interface that enables developers to access numerous computational engines.
When creating and delivering computer vision systems, software engineers must optimise computing efficiency, especially given how complicated and time-consuming computer vision and image processing pipelines are becoming. If software developers for computer vision and image processing aren't achieving your objective or desired frames per second rate.
To improve performance, they should take into account Programmable vision computer for highly optimised algorithms. When looking for highly optimised algorithms to boost performance, particularly to replace underperforming OpenCV algorithms in the processing pipeline, they should take Programmable vision computer into consideration.
Background subtraction, perspective warp, temporal noise reduction, histogram equalisation, and lens distortion are among the optimised Programmable vision computer methods.
Programmable vision computer allows programmers the freedom to create pipelines for computer vision and image processing. It provides Python bindings for the algorithms in addition to C support, enabling you to use Programmable vision computer directly in Python scripts.
Sl no | Topic |
1 | Market Segmentation |
2 | Scope of the report |
3 | Abbreviations |
4 | Research Methodology |
5 | Executive Summary |
6 | Introduction |
7 | Insights from Industry stakeholders |
8 | Cost breakdown of Product by sub-components and average profit margin |
9 | Disruptive innovation in the Industry |
10 | Technology trends in the Industry |
11 | Consumer trends in the industry |
12 | Recent Production Milestones |
13 | Component Manufacturing in US, EU and China |
14 | COVID-19 impact on overall market |
15 | COVID-19 impact on Production of components |
16 | COVID-19 impact on Point of sale |
17 | Market Segmentation, Dynamics and Forecast by Geography, 2023-2030 |
18 | Market Segmentation, Dynamics and Forecast by Product Type, 2023-2030 |
19 | Market Segmentation, Dynamics and Forecast by Application, 2023-2030 |
20 | Market Segmentation, Dynamics and Forecast by End use, 2023-2030 |
21 | Product installation rate by OEM, 2023 |
22 | Incline/Decline in Average B-2-B selling price in past 5 years |
23 | Competition from substitute products |
24 | Gross margin and average profitability of suppliers |
25 | New product development in past 12 months |
26 | M&A in past 12 months |
27 | Growth strategy of leading players |
28 | Market share of vendors, 2023 |
29 | Company Profiles |
30 | Unmet needs and opportunity for new suppliers |
31 | Conclusion |
32 | Appendix |