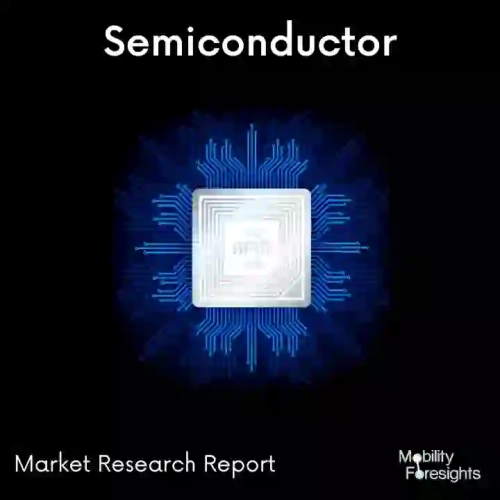
- Get in Touch with Us
Last Updated: Apr 25, 2025 | Study Period:
Artificial Intelligence (AI) is driving the next technology revolution and marks one of the most significant technological developments in recent years, with AI Systems on a Chip (SoC). AI SoCs are system-on-chip devices that have been purpose-built to handle the deep and complex calculations needed for machine learning or neural network processing, bringing AI computing closer so that decisions can be made where they matter at (or near) the data source.
This market is dominated by massive R&D investments from leading semiconductor companies like NVIDIA, Intel, and Qualcomm who are all pushing the boundaries of AI performance and efficiency. Increasing implementation of AI technologies in consumer electronics, automotive, healthcare, and other industrial products is one likely driver of the market.
Demand for edge computing solutions is a key factor underpinning the AI SoC market. IoT devices and other edge-connected systems are creating a massive amount of data which is resulting in the rise of the need for local processing capabilities to reduce latencies, leading to better response times.
AI SoCs allow these edge devices to field complex AI tasks, without an expensive and time-consuming trip back up to the callous cloud. It can be very useful for those applications where time is of the essence and decisions have to be taken like autonomous vehicles, industrial automation, or smart home devices.
In addition, the semiconductor manufacturing technology advances also drive the market. Further, shrinking process nodes to 7nm and even 5nm has helped also produce more high-performance, power-efficient AI SoCs. These smaller nodes incorporate larger transistor density, for improved performance and power saving.
AI SoCs are also adopting advancements in AI algorithms and architecture (e.g., convolutional neural networks, CNNs; recurrent neural networks, RNN) that will further optimize performance improvements to their AI processing engines as well. The advances in technology are broadening the use of AI to an increasing number of niches and environments.
As such, the AI market's state-of-the-art integrated SoC is expected to continue growth going forward given more extensive integration for them into everyday devices and systems. In addition, the new AI SoCs are just one part of an environment that is rapidly becoming more sophisticated and powerful with emerging technologies such as neuromorphic computing, quantum computing, etc.
Moreover, an increase in AI research and development initiatives, accompanied by rising investments from various governments & corporate investors is presumed to stimulate market growth. The increasing penetration of AI across various sectors coupled with growing living standards are responsible for the advanced AI SoCs market and creating a bright outlook.
Artificial Intelligence (AI) System on Chip (SoC), is a groundbreaking innovation in computing. Supporting this high-performance, power-efficient Manufacturing-on-the-Jet capability is an extension of NVIDIA's rich and diverse set of deep learning tools available to its OEM partners. AI SoCs are made to perform the intensive calculations needed for ML and NN processing, effectively enabling AI compute to reside at or near its data source. Closer means less latency and lower power, so AI SoCs are a good fit for edge computing applications where making decisions quickly is important.
These general-purpose architectures are composed of various components, including neural processing units (NPUs), digital signal processors (DSPs), and classical CPUs/GPUs. Each of these parts combines to improve the handling of AI workloads, like image classification or natural language processing and predictive analytics. Giving all of these components to work together in one chip alone reduces the physical size and complexity of hardware but also improves performance by reducing data transfer latencies from a processing unit that needs other parts.
Increasing demand for AI capabilities in consumer and industrial applications is one of the major factors driving the growth of AI SoC trans, Whether that is smartphones and smart speakers industrial robots, or autonomous drones - the need for powerful yet efficient AI processing has never been bigger.
The latter allows these devices to execute complex processes locally using AI SoCs, rather than continuously requiring communication with the cloud-based servers. The use of local processing is extremely important for high-speed applications, such as autonomous driving and advanced robotics, where any latency degrades performance.
The future of AI SoCsWhile the current landscape offers some promising solutions, continuous improvements will be expected in terms of performance and efficiency, driven by advancements in semiconductor technology as well as AI algorithms. Researchers and engineers are looking at new materials, design techniques, and fabrication methods to wring more power out of arm-wave SoCs.
Moreover, by further progressing its AI models and training techniques development, the chips are expected to become even more capable of handling increasingly complex applications.
The Global AI System on Chip (SoC) Market was valued at $XX Billion in 2023 and is projected to reach $XX Billion by 2030, reflecting a compound annual growth rate (CAGR) of XX% from 2024 to 2030.
Heterogeneous computing architectures
Heterogeneous computing architectures that consist of CPUs, GPUs, and dedicated AI accelerators- the key elements in an increasing number of new AI SoCs now find their way into single chips. This process is designed to maximize computational energy for AI workloads, meaning faster data processing and more complex output from an artificial intelligence model.
Edge AI Processing:
Edge-specific usage of AI processing is to imbue edge devices with their internal state machines so that they become capable of managing and controlling the flow in which data moves through them - reducing latency that can be caused by relaying information across networks up into cloud-based computational systems. On one end of the spectrum, edge AI processing enables applications to make real-time decisions and analytics like autonomous vehicles, IoT devices, or industrial automation that have overall lower system latency.
Neural Network Support
Designers of these AI SoCs are tailoring support for more complex neural network architectures, including deep-learning and convolutional neural network algorithms. With this capability, complex AI applications like natural language processing (i.e., intelligent assistants/chatbots) or computer vision can be executed with better efficiency and can benefit from higher performance.
Energy Efficient Renovations:
AI SoC design evolution techniques are implemented to match the need for more energy-efficient computing solutions while still providing high-performance levels. These energy-efficient AI SoCs support the deployment of artificial intelligence capabilities in power-constrained environments, such as mobile and sensor devices but at high levels of performance.
NVIDIA Orin:
Introducing the NVIDIA Orin - The Platform For High-Performance AI on Autonomous Machines for Robotics More than 200 TOPS (Tera Operations Per Second) of AI performance. This is a powerful SoC that can take high-end AI workloads which in turn allows it to process faster and make real-time decisions for autonomous systems.
Qualcomm Snapdragon 8cx Gen2:
Snapdragon 8cx Gen 3 SoC for AI and Harman-developed audio, Integrated with Snapdragon X65 modem fastest connectivity(optimizer-upto download speeds of over a Gigabit). This provides improved CPU and GPU performance for faster multitasking, as well as better AI inference. It is even more rapidly processed with 5G technology, which the second renders it a prominent AI contender for next-gen applications.
Apple M2:
The Apple M2 chip is equipped with an 8-core CPU and a 10-core GPU, faster than the previous generation. It features a 16-core Neural Engine for those serious machine learning jobs. Meanwhile, the M2 with its greater performance and efficiency helps enable demanding AI and computational workloads across different device types.
AMD Ryzen Embedded V3000:
Ryzen Embedded V3000 is a high-performance SoC for edge AI applications with integrated security and Multiple AI frameworks for flexible deployment The solid strong capabilities and security features make it ideal for applying to critical edge AI solutions in industrial, and commercial situations.
Sr.No | Topic |
1 | Market Segmentation |
2 | Scope of the report |
3 | Research Methodology |
4 | Executive Summary |
5 | Average B2B by price |
6 | Introduction |
7 | Insights from Industry stakeholders |
8 | Key Drivers for Global AI System on Chip (SoC) Market |
9 | Disruptive Innovation in the Industry |
10 | Overview of the Global AI System on Chip (SoC) Market |
11 | Major impact on Technological advancements |
12 | Consumer trends in the industry |
13 | Recent Technological Trends in Global AI System on Chip (SoC) Market |
14 | SWOT Analysis of Key Market Players |
15 | New product development in the past 12 months |
16 | Market Size, Dynamics, and Forecast by Geography, 2024-2030 |
17 | Market Size, Dynamics, and Forecast by Type, 2024-2030 |
18 | Market Size, Dynamics, and Forecast by End User, 2024-2030 |
19 | Market Size, Dynamics, and Forecast by Application, 2024-2030 |
20 | Competitive landscape |
21 | Gross margin and average profitability of suppliers |
22 | Merger and Acquisition in the past 12 months |
23 | Growth strategy of leading players |
24 | Market share of vendors, 2023 |
25 | Market Company Profiles |
26 | Unmet needs and opportunities for new suppliers |
27 | Conclusion |