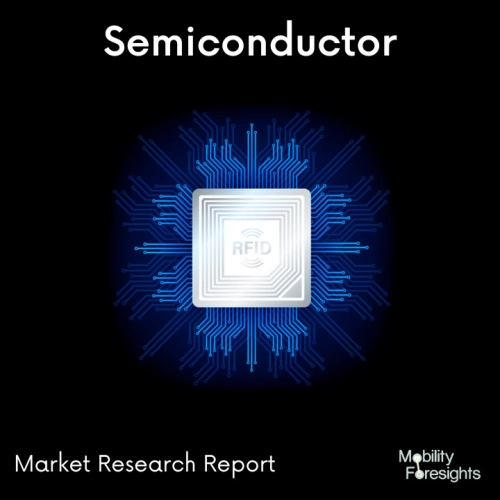
- Get in Touch with Us
Last Updated: Apr 25, 2025 | Study Period: 2024-2030
The computational challenge of creating or updating a map of an uncharted environment while concurrently tracking an agent's location inside it is known as simultaneous localization and mapping (SLAM) image sensor.
Although this seems like a chicken-and-egg issue at first, there are various algorithms that can solve it in a particular context in at least somewhat tractable time. The particle filter, extended Kalman filter, covariance intersection, and GraphSLAM are examples of popular approximation techniques.
Robot navigation, robotic mapping, and odometry for virtual reality or augmented reality all make use of SLAM algorithms, which are based on ideas in computational geometry and computer vision.
The goal of SLAM algorithms is operational compliance rather than perfection and they are adapted to the resources that are available. Self-driving cars, unmanned aerial vehicles, autonomous underwater vehicles, planetary rovers, more recent domestic robots, and even inside the human body use published methods.
The SLAM challenge is to calculate an estimate of the agent's state (display style x t x t) and a map of the environment (display style m t m t) from a set of controls (display style u t u t) and sensor observations (display style o t o t) over discrete time steps (display style t t).
The following equations are approximated using statistical methods such as Kalman filters and particle filters. For the robot's pose and the map's parameters, they offer an assessment of the posterior probability distribution.
In order to reduce algorithmic complexity for large-scale applications, techniques that conservatively approximate the aforementioned model using covariance intersection are able to avoid reliance on statistical independence assumptions. Other approximation techniques use straightforward bounded-region representations of uncertainty to increase computational efficiency.
The Global simultaneous localization and mapping (SLAM) image sensor market accounted for $XX Billion in 2023 and is anticipated to reach $XX Billion by 2030, registering a CAGR of XX% from 2024 to 2030.
The OGOVE global shutter (GS) image sensor simultaneous localization and mapping (SLAM) image sensor, a small-form-factor, high-sensitivity device for AR/VR/MR, the metaverse, drones, machine vision, and barcode scanner products, was announced by OMNIVISION, a leading global developer of semiconductor solutions, including advanced digital imaging, analogue, and touch & display technology.
Compared to the previous generation, this image sensor is 26% smaller and uses more than 50% less power.
Based on OMNIVISION's OmniPixel 3-GS technology, the 3.0-micron pixel on the OG0VE CMOS image sensor is highly sensitive. The sensor can be utilised for any application requiring simultaneous localization and mapping (SLAM), gesture detection, head and eye tracking, depth and motion detection due to its global shutter pixel architecture and exceptional low-light sensitivity.
It is only 3.6mm by 2.7mm in size, has a 640 Ã 480 resolution, and uses a 1/7.5-inch optical format. At 60 frames per second (fps) with VGA, it uses less than 34mW of electricity, which is extremely low.
Sl no | Topic |
1 | Market Segmentation |
2 | Scope of the report |
3 | Abbreviations |
4 | Research Methodology |
5 | Executive Summary |
6 | Introduction |
7 | Insights from Industry stakeholders |
8 | Cost breakdown of Product by sub-components and average profit margin |
9 | Disruptive innovation in the Industry |
10 | Technology trends in the Industry |
11 | Consumer trends in the industry |
12 | Recent Production Milestones |
13 | Component Manufacturing in US, EU and China |
14 | COVID-19 impact on overall market |
15 | COVID-19 impact on Production of components |
16 | COVID-19 impact on Point of sale |
17 | Market Segmentation, Dynamics and Forecast by Geography, 2024-2030 |
18 | Market Segmentation, Dynamics and Forecast by Product Type, 2024-2030 |
19 | Market Segmentation, Dynamics and Forecast by Application, 2024-2030 |
20 | Market Segmentation, Dynamics and Forecast by End use, 2024-2030 |
21 | Product installation rate by OEM, 2023 |
22 | Incline/Decline in Average B-2-B selling price in past 5 years |
23 | Competition from substitute products |
24 | Gross margin and average profitability of suppliers |
25 | New product development in past 12 months |
26 | M&A in past 12 months |
27 | Growth strategy of leading players |
28 | Market share of vendors, 2023 |
29 | Company Profiles |
30 | Unmet needs and opportunity for new suppliers |
31 | Conclusion |
32 | Appendix |