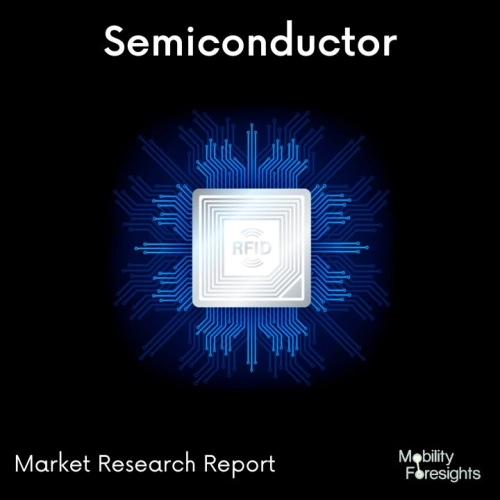
- Get in Touch with Us
Last Updated: Apr 25, 2025 | Study Period:
The integration of artificial intelligence (AI) into vision processors is enabling more sophisticated and intelligent vision-based applications. AI algorithms are empowering vision processors to perform tasks such as object recognition, image segmentation, and anomaly detection, leading to increased adoption in various industries.
The demand for smart devices, such as smartphones, wearable electronics, and smart home appliances, is driving the need for compact and efficient vision processors. These devices require real-time processing of visual data for features such as facial recognition, augmented reality, and gesture recognition.
The rapid growth of visual data from sources such as surveillance cameras, drones, and social media is creating a demand for powerful and scalable vision processing solutions. Vision processors are essential for analyzing and extracting meaningful insights from this vast amount of visual data.
To meet the size and power constraints of smart devices and wearable electronics, vision processor manufacturers are focusing on miniaturization and integration. This trend is leading to the development of smaller and more powerful vision processors that can be easily embedded into these devices.
To handle the diverse computational demands of vision processing applications, heterogeneous architectures are becoming increasingly prevalent. These architectures combine different processing elements, such as CPUs, GPUs, and specialized accelerators, to optimize performance and energy efficiency for specific tasks.
Cloud-based vision processing services are emerging as a valuable option for applications that require high-performance processing or access to specialized expertise. These services allow users to offload computationally intensive tasks to the cloud, enabling real-time analysis of large volumes of visual data.
As vision processors collect and process increasingly sensitive visual data, security and privacy concerns are becoming paramount. Vision processor manufacturers are integrating robust security measures and privacy-enhancing techniques to protect user data and prevent unauthorized access.
The future of the global vision processor market is brimming with promising opportunities. The increasing demand for smart devices, the proliferation of visual data, and the continuous advancements in AI are expected to drive significant market growth in the coming years.
A vision processor is a specialized hardware component designed for processing and analyzing visual data, typically in the form of images or video streams. It is a key component in computer vision systems, enabling the extraction of meaningful information and insights from visual inputs.
Types
Application
Benefits
Challenges in Vision Processors Market
It is one of several specialist processors, like the GPU, which are typically helpful in machine learning. It is a specialised processor designed to handle tasks like image processing.The vision processing unit is better suited for executing various machine vision algorithms. These tools are made for parallel processing and can be built with specific resources for acquiring visual data from cameras. It can process visual data intelligently to assist develop smart, power-efficient visual data solutions.
Miniaturization and Integration: The increasing demand for compact and lightweight devices in Vision Processors Market, particularly in wearable electronics and autonomous systems, is driving the miniaturization of vision processors. This trend is being facilitated by advancements in semiconductor technology, enabling the integration of more powerful and sophisticated vision processing capabilities into smaller form factors.
Edge Computing and AI Integration: Edge computing is gaining traction in vision processing applications, bringing AI capabilities closer to the data source and reducing latency. This enables real-time processing of visual data, enhancing the responsiveness and efficiency of vision-based systems. The integration of AI algorithms into vision processors is further augmenting their capabilities, enabling tasks such as object recognition, scene understanding, and anomaly detection.
Heterogeneous Computing Architectures: To meet the diverse computational demands of vision processing applications, heterogeneous architectures are becoming increasingly prevalent. These architectures combine different processing elements, such as CPUs, GPUs, and specialized accelerators, to optimize performance and energy efficiency for specific tasks.
Sensor Fusion and Multimodal Processing: Vision processors are increasingly integrating with other sensors, such as lidar, radar, and inertial measurement units (IMUs), to provide a more comprehensive understanding of the environment. This sensor fusion enables more robust and accurate perception, particularly in complex and dynamic environments.
Cloud-Based Vision Processing and Services: Cloud-based vision processing services are emerging as a valuable option for applications that require high-performance processing or access to specialized expertise. These services allow users to offload computationally intensive tasks to the cloud, enabling real-time analysis of large volumes of visual data.
These trends indicate the evolving landscape of the global vision processor market, emphasizing the convergence of miniaturization, AI integration, heterogeneous architectures, sensor fusion, and cloud-based solutions. As vision processing technology continues to advance, it is poised to play an increasingly crucial role in various industries, including automotive, robotics, healthcare, and security.
To build on innovations that advance intelligence at the edge, Texas Instruments (TI)introduced a new family of six Arm® Cortex®-based vision processors that allow designers to add more vision and artificial intelligence (AI) processing at a lower cost, and with better energy efficiency, in applications such as video doorbells, machine vision and autonomous mobile robots.
This new family, which includes the AM62A, AM68A and AM69A processors, is supported by open-source evaluation and model development tools, and common software that is programmable through industry-standard application programming interfaces (APIs), frameworks and models. This platform of vision processors, software and tools helps designers easily develop and scale edge AI designs across multiple systems while accelerating time to market.
With 1,024 MACs, the newARC NPX6-1K NPU IPprovides a good entry point for designers to add support for the latest neural networksâespecially transformersâto power- and area-constrained vision-processing designs. Another version, the ARC NPX-1KFS NPU IP, provides state-of-the-art hardware safety features to accelerate ISO 26262 certification for automotive designs.
The NPX6-1K and 1KFS processors can be tightly integrated with theSynopsys ARC VPX2 DSP Processor IP, to produce the marketâs most area- and power-efficient AI + DSP solution for DSP and neural network transformers. The combined NPX6 and VPX solutions can scale upwards and configurations can be mixed and matched to support a large DSP with small AI, large AI, small DSP, etc.
The S32V234 is a 64-bit Arm Cortex-A53 S32V processor and a member of the second-generation vision processor family. The S32V234 processor has an image signal processor, a potent 3D graphic processing unit, dual APEX-2 vision accelerators, automotive-grade reliability, and functional safety & security features.
Comprehensive enablement for the S32V234 processor is supported by S32 Design Studio IDE for Vision, which includes a compiler, debugger, Vision SDK, Linux BSP, and graph tools.
For edge applications that require a lot of artificial intelligence (AI), Synopsys has introduced its newest generation of embedded vision processors with deep neural network (DNN) accelerator, giving what it claims is an industry-leading 35 TOPS (tera operations per second) performance.
New Embedded Vision Processors from Synopsys have been launched. With its deep neural network (DNN) accelerator, it promises to deliver 35 TOPS (tera operations per second), an industry-leading speed for edge applications that require a lot of artificial intelligence (AI).
The development of automotive radar/lidar, sensor system on chip (SoC), and advanced driver assist systems (ADAS) is also made possible by the introduction of a functional safety processor version. The new DesignWare ARC EV7x vision processors are based on the ARCv2 RISC instruction set architecture.
They have a heterogeneous architecture that integrates vector DSP, vector FPU, and neural network accelerator to support a range of intelligent consumer and automotive applications with built-in AES encryption.
Under typical settings, the optional DNN accelerator scalable from 880 to 14,080 MACs to allow a system to give up to 35 TOPS performance in 16-nanometer (nm) FinFET process technologies, which is four times the performance of the ARC EV6x processors from the previous generation.
The EV7x processors are an optimization of the EV6x processors, increasing the number of MACs was the simple way to scale the performance of a CNN (convolutional neural network) graph, but that increasing memory bandwidth needed for external memory accesses is actually more important in order to reduce power consumption. The new CPUsâ bandwidth management makes it possible.
A CNN graph must also be divided over rising MACs using sophisticated graph mapping methods. This causes the EV7x processors to accelerate frame-per-second throughput by up to 65% when compared to EV6x.
The multicore architecture of the new EV7x vision processors includes up to four high-performance vector processing units (VPUs), each of which includes a 32-bit scalar unit and a 512-bit wide vector DSP, configurable for 8-, 16-, or 32-bit operations to perform simultaneous multiply-accumulates on various data streams.
According to Synopsys, the DNN accelerator uses a unique design for faster memory access, more performance, and better power economy than alternative neural network IP.
The Rise of Intelligent Edge Devices with AI Acceleration. AI is not a recent development, and every one of us uses it daily to improve many facets of our lives.Edge computing, which offers possibilities to transfer AI workloads from the Intelligent Cloud to the Intelligent Edge for better reaction times and bandwidth savings, is the driving force behind this movement.
There is a significant trend to use AI/ML analytics in conjunction with Digital Twins and IoT in manufacturing as well as other sectors to gain better and quicker insights for enhanced Predictive Maintenance and other applications. When it comes to computer vision models that use huge data streams like photos or live video as input, the advantage of edge installations is particularly great.
Since edge computing allows for local processing at the device or client, feeding big data streams onto a cloud data centre is no longer necessary and poses no privacy risks. Computer vision and deep learning techniques can be used by edge video analytics systems either directly inside the camera or in conjunction with an external edge computing system.
The computer vision AI models are often sent to an edge device after being pre-trained in the cloud. In this method, the resource-intensive training phase makes use of the power and scalability of the Cloud, while real-time model inference and assessment make use of the Edgeâs low latency.
This is made even more pronounced by the development of specialised silicon created and constructed to speed AI workloads, such as Neural Processing Unit (NPU), Tensor Processing Unit (TPU), Vision Processing Unit (VPU), and other specific AI accelerator chips. A period similar to the 1990s, when the widespread use of specialised graphics cards (GPUs) sparked a boom in 3D computer graphics, is again upon us.
The commoditization of AI-acceleration processors like NPUs, TPUs, and comparable ones in edge devices is expected to lead to similar outcomes. In our Summer of AI 2021 , discussed Edge Computing and (Video) Analytics and showed off a straightforward but effective edge AI example for workplace safety using no-code training and a Raspberry Pi with Adafruit for edge execution.
Edge AI was mentioned in the Top Trends 2022 piece as another continuing technology to pay special attention to. In this article, we will discuss some of the more recent products and services that were unveiled at Build as well as some Edge .
AI trends that are based on research that is urging business leaders to start using this cutting-edge technology into their operational procedures in order to meet the changing demands of their clients and workforce.
The North American market, particularly the USA, will be one of the prime markets for (Vision Processors Market) due to the nature of industrial automation in the region, high consumer spending compared to other regions, and the growth of various industries, mainly AI, along with constant technological advancements. The GDP of the USA is one of the largest in the world, and it is home to various industries such as Pharmaceuticals, Aerospace, and Technology.
The average consumer spending in the region was $72K in 2023, and this is set to increase over the forecast period. Industries are focused on industrial automation and increasing efficiency in the region. This will be facilitated by the growth in IoT and AI across the board. Due to tensions in geopolitics, much manufacturing is set to shift towards the USA and Mexico, away from China. This shift will include industries such as semiconductors and automotive.
The European market, particularly Western Europe, is another prime market for (Vision Processors Market) due to the strong economic conditions in the region, bolstered by robust systems that support sustained growth. This includes research and development of new technologies, constant innovation, and developments across various industries that promote regional growth.
Investments are being made to develop and improve existing infrastructure, enabling various industries to thrive. In Western Europe, the margins for (Vision Processors Market) are higher than in other parts of the world due to regional supply and demand dynamics. Average consumer spending in the region was lower than in the USA in 2023, but it is expected to increase over the forecast period.
Eastern Europe is anticipated to experience a higher growth rate compared to Western Europe, as significant shifts in manufacturing and development are taking place in countries like Poland and Hungary. However, the Russia-Ukraine war is currently disrupting growth in this region, with the lack of an immediate resolution negatively impacting growth and creating instability in neighboring areas. Despite these challenges, technological hubs are emerging in Eastern Europe, driven by lower labor costs and a strong supply of technological capabilities compared to Western Europe.
There is a significant boom in manufacturing within Europe, especially in the semiconductor industry, which is expected to influence other industries. Major improvements in the development of sectors such as renewable energy, industrial automation, automotive manufacturing, battery manufacturing and recycling, and AI are poised to promote the growth of (Vision Processors Market) in the region.
Asia will continue to be the global manufacturing hub for (Vision Processors Market) over the forecast period with China dominating the manufacturing. However, there will be a shift in manufacturing towards other Asian countries such as India and Vietnam. The technological developments will come from China, Japan, South Korea, and India for the region.
There is a trend to improve the efficiency as well as the quality of goods and services to keep up with the standards that are present internationally as well as win the fight in terms of pricing in this region. The demand in this region will also be driven by infrastructural developments that will take place over the forecast period to improve the output for various industries in different countries.
There will be higher growth in the Middle East as investments fall into place to improve their standing in various industries away from petroleum. Plans such as Saudi Arabia Vision 2030, Qatar Vision 2030, and Abu Dhabi 2030 will cause developments across multiple industries in the region.
There is a focus on improving the manufacturing sector as well as the knowledge-based services to cater to the needs of the region and the rest of the world. Due to the shifting nature of fossil fuels, the region will be ready with multiple other revenue sources by the time comes, though fossil fuels are not going away any time soon.
Africa is expected to see the largest growth in (Vision Processors Market) over the forecast period, as the region prepares to advance across multiple fronts. This growth aligns with the surge of investments targeting key sectors such as agriculture, mining, financial services, manufacturing, logistics, automotive, and healthcare.
These investments are poised to stimulate overall regional growth, creating ripple effects across other industries as consumer spending increases, access to products improves, and product offerings expand. This development is supported by both established companies and startups in the region, with assistance from various charitable organizations.
Additionally, the presence of a young workforce will address various existing regional challenges. There has been an improvement in political stability, which has attracted and will continue to attract more foreign investments. Initiatives like the African Continental Free Trade Area (AfCFTA) are set to facilitate the easier movement of goods and services within the region, further enhancing the economic landscape.
Latin America and the Oceania region will showcase growth over the forecast period in (Vision Processors Market). In Latin America, the focus in the forecast period will be to improve their manufacturing capabilities which is supported by foreign investments in the region. This will be across industries mainly automotive and medical devices.
There will also be an increase in mining activities over the forecast period in this region. The area is ripe for industrial automation to enable improvements in manufacturing across different industries and efficiency improvements. This will lead to growth of other industries in the region.
Margin Comparison (Highest to lowest) | Region | Remarks |
1 | Europe | The supply chain demands and the purchasing power in the region enable suppliers to extradite a larger margin from this region than other regions. This is for both locally manufactured as well as imported goods and services in the region. |
2 | North America | Due to the high spending power in this region, the margins are higher compared to the rest of the world, but they are lower than Europe as there is higher competition in this region. All the suppliers of goods and services target USA as a main market thereby decreasing their margins compared to Europe |
3 | Asia | Lower purchasing power, coupled with higher accessibility of services in this regions doesnât enable suppliers to charge a high margin making it lower than Europe and North America. The quality of goods and services are also affected due to this aspect in the region |
4 | Africa and ROW | The margins are the lowest in this region, except for Australia and New Zealand as the countries in this region donât have much spending power and a large portion of the products and services from this area is exported to other parts of the world |
The Global Vision Processors market can be segmented into following categories for further analysis.
Here is a list of some of the leading Vision Processor companies in the world:
SI No. | Topic |
1 | Scope Of the Report |
2 | Market Segmentation |
3 | Research Methodology |
4 | Executive Summary |
5 | Key Predictions for Vision Processor Market |
6 | Insight From Industry Stakeholders |
7 | Global Vision Processor Market- Overview |
8 | Global Vision Processor Manufacturer’s Footprint |
9 | Average B--B Price of Vision Processor, by Region |
10 | Recent Developments in Vision Processor Market |
11 | Major Drivers for Vision Processor |
12 | Opportunities For Vision Processor in Different Industries |
13 | Industrial Supply Chain for Vision Processor |
14 | Increasing demand for Vision Processor |
15 | Growing adoption of Vision Processor in new applications |
16 | Market Size, Dynamics, and Forecast by Geography (2024-2030) |
17 | Market Size, Dynamics And Forecast by Application (2024-2030) |
18 | Market Size, Dynamics And Forecast by End Users (2024-2030) |
19 | Growth Strategies Of Major Players |
20 | Competitive Landscape |
21 | Recent Mergers And Acquisitions In Past Years |
22 | Market Share Of Industry Players - 2023 |
23 | Company Profiles |
24 | Conclusion |