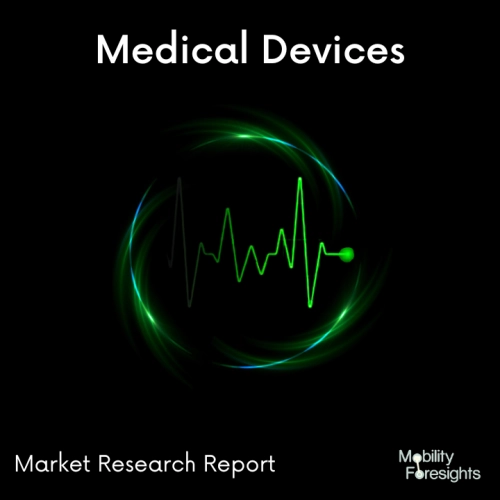
- Get in Touch with Us
Last Updated: Apr 25, 2025 | Study Period: 2023-2030
The Wearable Concussion Sensor is a revolutionary device designed to help detect and prevent concussions.It is a small, lightweight, and unobtrusive device worn on the head underneath a helmet. The sensor is designed to detect signs of a concussion and alert coaches, trainers, and medical personnel if a potential injury has occurred.
The device works by detecting the head movement associated with a concussion and using an internal accelerometer to measure the force of the impact. It then sends an alert to the wearer and any medical personnel nearby, allowing them to take the appropriate action.
The Wearable Concussion Sensor has the potential to revolutionise the way concussions are managed.It provides a more objective method of detecting and preventing concussions, rather than relying on subjective reports from athletes or coaches.
It can also provide data to help medical personnel better understand the cause and effects of concussions.The Wearable Concussion Sensor is a valuable tool for athletes of all ages, from youth sports to professional leagues.
It can help improve safety for athletes and reduce the risk of long-term health problems associated with concussions.The device is also relatively affordable, making it accessible to a wider range of athletes and teams.
The Wearable Concussion Sensor is a revolutionary device that has the potential to revolutionise the way concussions are managed.It can help improve safety for athletes and reduce the risk of long-term health problems associated with concussions. With its easy-to-use design and affordable price, the sensor is a valuable tool for athletes of all ages and levels.
The Global Wearable Concussion Sensor market accounted for $XX Billion in 2022 and is anticipated to reach $XX Billion by 2030, registering a CAGR of XX% from 2023 to 2030.
With sensitivity and reproducibility higher than clinical rating scales for concussions and a wide range of other neurological diseases, wearable motion sensors have displayed potential for use in the development of concussion gait and balance biomarkers.
Thus, one extremely promising avenue for enhancing the current clinical options for concussion diagnosis and management is the application of machine learning to wearable motion sensor data classification.
While gyroscopes and accelerometers are commonly found in wearable motion sensors, accelerometers are far more power-efficient than gyroscopes. The phybrata sensor, for example, uses a single accelerometer, which makes it more suitable for long-term activity monitoring.
The affordability, user-friendliness, and remote patient monitoring compatibility of wearable sensors make them particularly interesting. The most useful metrics to identify the various physiological system deficits that can arise from concussions and other neurological diseases are balance and gait assessments, among the many other physiological metrics that can now be assessed using wearable devices.
The reason for its usefulness is because concussion injuries can cause widespread changes to the brain, vestibular system, visual system, or spinal cord, which can impair balance and movement.
The central integration of important sensory system inputs is compromised when the main brain regions involved in sensory integrationâsuch as the brainstem and cerebellumâare involved.
Both spinal cord disturbances and problems with the visual system further impede visual and somatosensory inputs. Cognitive decline is also associated with difficulties in balance and gait. Unfortunately, access to gold-standard laboratory research instruments like instrumented gait analysis for gait assessment and computerised dynamic posturography for balance assessment is limited to a relatively small number of concussion specialists and patients.
In the last ten years, wearable motion sensors have attracted a lot of attention from researchers interested in assessing balance and gait abnormalities linked to concussions because of their restricted access.
The management of numerous chronic medical illnesses could be substantially improved by remote patient monitoring with the help of a wearable device that can classify and quantify various physiological deficits without relying on the cloud.
Sl no | Topic |
1 | Market Segmentation |
2 | Scope of the report |
3 | Abbreviations |
4 | Research Methodology |
5 | Executive Summary |
6 | Introduction |
7 | Insights from Industry stakeholders |
8 | Cost breakdown of Product by sub-components and average profit margin |
9 | Disruptive innovation in the Industry |
10 | Technology trends in the Industry |
11 | Consumer trends in the industry |
12 | Recent Production Milestones |
13 | Component Manufacturing in US, EU and China |
14 | COVID-19 impact on overall market |
15 | COVID-19 impact on Production of components |
16 | COVID-19 impact on Point of sale |
17 | Market Segmentation, Dynamics and Forecast by Geography, 2023-2030 |
18 | Market Segmentation, Dynamics and Forecast by Product Type, 2023-2030 |
19 | Market Segmentation, Dynamics and Forecast by Application, 2023-2030 |
20 | Market Segmentation, Dynamics and Forecast by End use, 2023-2030 |
21 | Product installation rate by OEM, 2023 |
22 | Incline/Decline in Average B-2-B selling price in past 5 years |
23 | Competition from substitute products |
24 | Gross margin and average profitability of suppliers |
25 | New product development in past 12 months |
26 | M&A in past 12 months |
27 | Growth strategy of leading players |
28 | Market share of vendors, 2023 |
29 | Company Profiles |
30 | Unmet needs and opportunity for new suppliers |
31 | Conclusion |
32 | Appendix |